I have several images for which i need to do OMR by detecting checkboxes using computer vision.
I'm using findContours to draw contours only on the checkboxes in scanned document. But the algorithm extracts each and every contours of the text.
from imutils.perspective import four_point_transform
from imutils import contours
import numpy as np
import argparse, imutils, cv2, matplotlib
import matplotlib.pyplot as plt
import matplotlib.image as mpimg
image = cv2.imread("1.jpg")
gray = cv2.cvtColor(image, cv2.COLOR_BGR2GRAY)
blurred = cv2.GaussianBlur(gray, (5, 5), 0)
edged = cv2.Canny(blurred, 75, 200)
im_test = [blurred, cv2.GaussianBlur(gray, (7, 7), 0), cv2.GaussianBlur(gray, (5, 5), 5), cv2.GaussianBlur(gray, (11, 11), 0)]
im_thresh = [ cv2.threshold(i, 127, 255, 0) for i in im_test ]
im_thresh_0 = [i[1] for i in im_thresh ]
im_cnt = [cv2.findContours(thresh, cv2.RETR_TREE, cv2.CHAIN_APPROX_SIMPLE)[0] for thresh in im_thresh_0]
im_drawn = [cv2.drawContours(image.copy(), contours, -1, (0,255,0), 1) for contours in im_cnt]
plt.imshow(im_drawn[0])
plt.show()
Input Image: 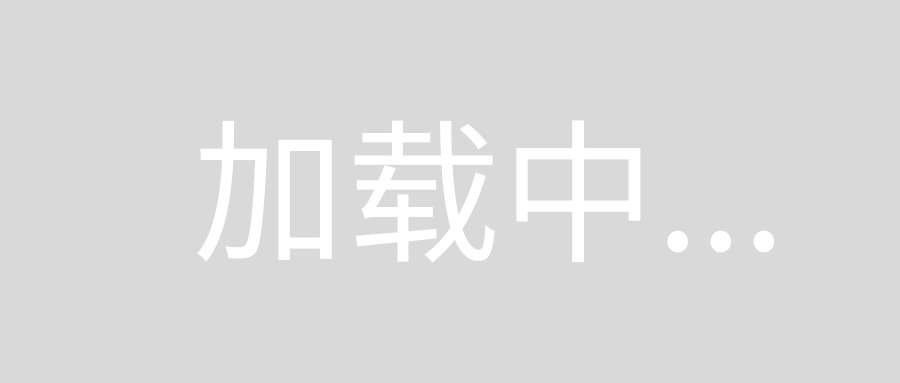
Since we only want to detect checkboxes, the idea is to use two filtering methods to isolate the desired boxes from the words. After preprocessing and finding the contours, we can iterate through each contour and apply the filters. We use cv2.contourArea()
with minimum and maximum threshold levels and then calculate the aspect ratio using cv2.approxPolyDP()
since a square will have an aspect ratio close to 1.
To detect edges in the image, we can use cv2.Canny()
and then grab contours using cv2.findContours()
which results in this image. Notice how all contours including words and checkboxes were detected.
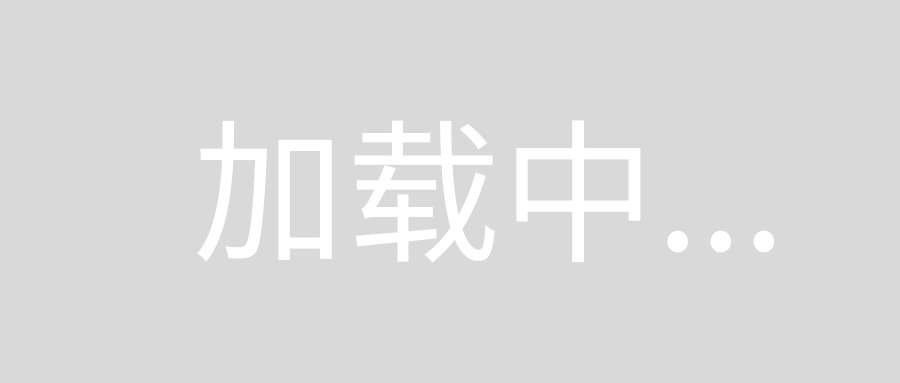
Next we iterate through each detected contour and filter using the threshold area and the aspect ratio. Using this method, all 52 checkboxes were detected.
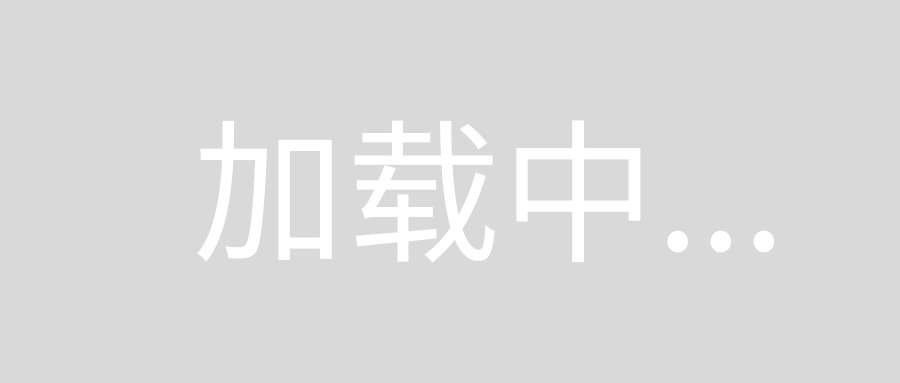
Output
('checkbox_contours', 52)
To prevent potential false positives, we can add a 3rd filter to ensure that each contour has four points (higher chance it is a square). If the input image was from an angle, we can use a four point transform as a preprocessing step to obtain a birds eye view of the image.
Another input image set
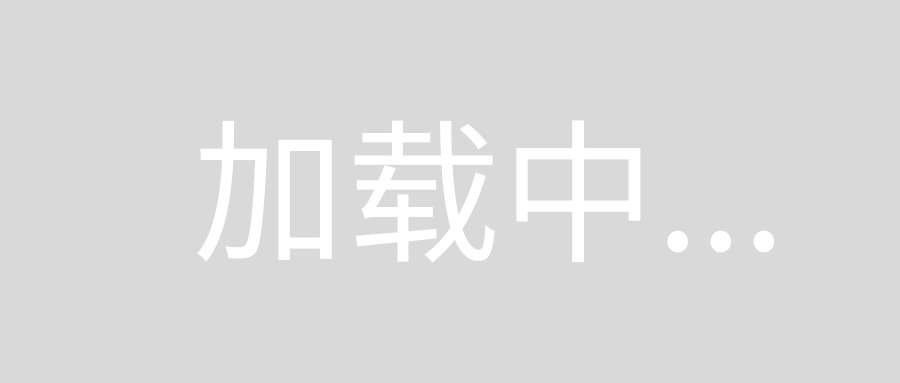
Output
('checkbox_contours', 2)
Code
import numpy as np
import imutils, cv2
original_image = cv2.imread("1.jpg")
image = original_image.copy()
gray = cv2.cvtColor(image, cv2.COLOR_BGR2GRAY)
blurred = cv2.GaussianBlur(gray, (5, 5), 0)
edged = cv2.Canny(blurred, 120, 255, 1)
cv2.imshow("edged", edged)
cnts = cv2.findContours(edged.copy(), cv2.RETR_EXTERNAL, cv2.CHAIN_APPROX_SIMPLE)
cnts = imutils.grab_contours(cnts)
checkbox_contours = []
threshold_max_area = 250
threshold_min_area = 200
contour_image = edged.copy()
for c in cnts:
peri = cv2.arcLength(c, True)
approx = cv2.approxPolyDP(c, 0.035 * peri, True)
(x, y, w, h) = cv2.boundingRect(approx)
aspect_ratio = w / float(h)
area = cv2.contourArea(c)
if area < threshold_max_area and area > threshold_min_area and (aspect_ratio >= 0.9 and aspect_ratio <= 1.1):
cv2.drawContours(original_image,[c], 0, (0,255,0), 3)
checkbox_contours.append(c)
print('checkbox_contours', len(checkbox_contours))
cv2.imshow("checkboxes", original_image)
cv2.waitKey(0)
Well... Are the checkboxes always in that region of the image? The checkboxes Always maintain the same size area on the image?
If yes, you can run findContours only in that region of the image...
Or maybe the Template Matching with Multiple Objects, example from OpenCV docs: https://docs.opencv.org/3.4.3/d4/dc6/tutorial_py_template_matching.html